
● Market risk analysis using extreme value theory and time series models
● Credit scoring with sparse regularized regression
● Financial Market Analysis with Text Mining
● Statistical modeling to measure credit risk
● Development of a Rental Real Estate Income Forecasting Model
● Modified LASSO estimators for nonlinear regression models with long-memory
disturbances
■ Bayesian Financial Time Series Analysis
We are engaged in quantitative analysis of financial time series usingBayesian statistics. By employing Bayesian statistics, we can achievea unified interpretation of results and gain deep insights intopatterns and dynamics of financial time series. However, the use ofBayesian statistics increases computational difficulties. Wecontribute to the progress of financial analysis by enhancingcomputational accuracy and efficiency through mathematical analysisand the development of novel techniques, such as scalablecomputational methods using piecewise deterministic Markov processes.
■ Market risk management combining time
series models and
extreme value theory
Calculation of daily correlation coefficient from high-frequency data
In market risk management, the risk of extremely large losses due to changes in asset prices is identified and used for forecasting, for example, by estimating the quantile corresponding to the upper 1% point of the loss distribution. Typically, extrapolated forecasts are made by fitting a time series model such as the GARCH model to the loss rate. Furthermore, the combined use of extreme value theory allows for more accurate high quantile point forecasts and enhances the statistical model’s ability to respond to the rare event of a large loss.
■ Corporate credit risk assessment with multiple integrated
databases
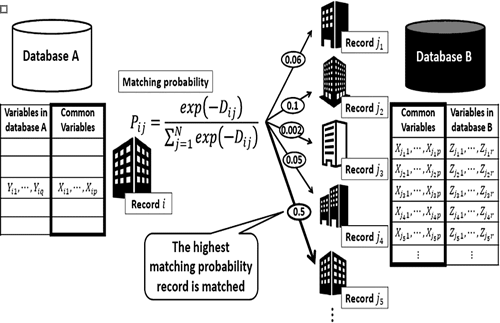
Banks have created statistical models and evaluated the creditworthiness of companies based on their own collected data on the repayment capacities of businesses. However, a variety of data have become available for use, such as online information, government statistics, and data from credit research companies, making it essential to integrate a number of databases. We are searching for practical ways to address the many issues that arise during database integration, such as probabilistic name-based aggregation methods, imputation of missing fields, and parameter estimation that consider data accuracy.

